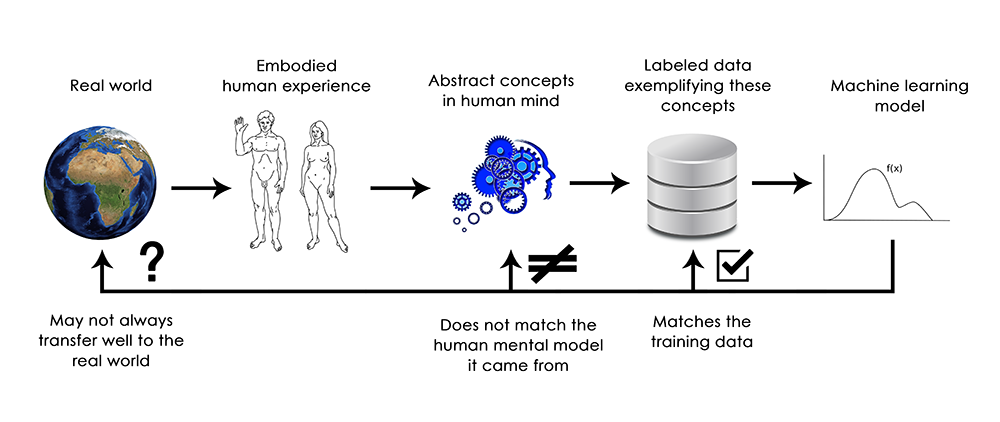
Deep learning has been hailed as a game-changer in the world of artificial intelligence (AI). With its ability to analyze vast amounts of data and improve its accuracy over time, deep learning has been used for a variety of applications, including image and speech recognition, natural language processing, and even self-driving cars.
However, like any technology, deep learning has its limitations. In this blog post, we will explore some of the most significant limitations of deep learning and why they matter.
The Black Box Problem
One of the most significant limitations of deep learning is the “black box” problem. Deep learning algorithms work by creating complex mathematical models that are trained on large datasets. While these models can accurately predict outcomes, it can be challenging to understand how they arrived at those predictions.
This lack of transparency can be problematic, especially in applications where human lives are at stake. For example, if a self-driving car’s deep learning model makes a mistake, it may not be clear why it happened, making it difficult to prevent it from happening again.
Overfitting
Another limitation of deep learning is overfitting. Overfitting occurs when a model is too complex and fits the training data too closely, causing it to perform poorly on new data. Deep learning models are particularly prone to overfitting because they are complex and have many parameters.
To prevent overfitting, deep learning models must be carefully designed and trained on large, diverse datasets. However, even with these precautions, overfitting can still occur, limiting the accuracy of deep learning models.
Lack of Contextual Understanding
Deep learning models are excellent at pattern recognition and can accurately identify objects, recognize speech, and translate languages. However, they lack contextual understanding, which can limit their effectiveness in certain applications.
For example, a deep learning model may accurately recognize a stop sign but may not understand the context of the sign, such as the need to stop at an intersection.
Data Requirements
Deep learning models require vast amounts of data to be trained effectively. This requirement can be a significant limitation in applications where data is scarce or difficult to obtain.
For example, in the medical field, it can be challenging to obtain large, diverse datasets that are necessary to train deep learning models effectively. This limitation can make it difficult to use deep learning in medical applications, such as disease diagnosis.
Computationally Intensive
Deep learning models are computationally intensive and require powerful hardware to train and run effectively. This requirement can be a significant limitation for organizations with limited computing resources.
Additionally, the energy consumption of deep learning models can be significant, making them environmentally unfriendly in some cases.
Conclusion
Deep learning has revolutionized the field of artificial intelligence and has enabled significant advances in a variety of applications. However, it is essential to understand the limitations of deep learning and how they can impact its effectiveness.
The black box problem, overfitting, lack of contextual understanding, data requirements, and computational intensity are all significant limitations of deep learning that must be overcome for it to reach its full potential. By understanding these limitations, we can develop better deep learning models and use them more effectively in real-world applications.
- Why Can’t I Make Create A New Folder on External Drive on Mac – Solved - April 28, 2024
- Tips on How to Become a DevOps Engineer - April 28, 2024
- Computer Programming Education Requirements – What You Need to Know - April 28, 2024