DataOps is a collaborative data management practice aimed at improvising the communication, integration, and automation of data flows between data managers and data consumers across an organization.
DataOps had begun as a set of best practices, it has now matured to become a new and independent approach to data analytics.

History of DataOps
- On 19 June 2014, DataOps was introduced by Lenny Liebmann, Contributing Editor, Information Week, in a blog post on the IBM Big Data & Analytics Hub titled ‘Three reasons why DataOps is essential for big data success’.
- The DataOps had been popularized by Andy Palmer of Tamr and Steph Locke.
- Gartner named DataOps on the Hype Cycle for Data Management in 2018.
- DataOps started gaining traction in 2017 by successfully bringing a DevOps approach to data.
Key challenges for Dataops
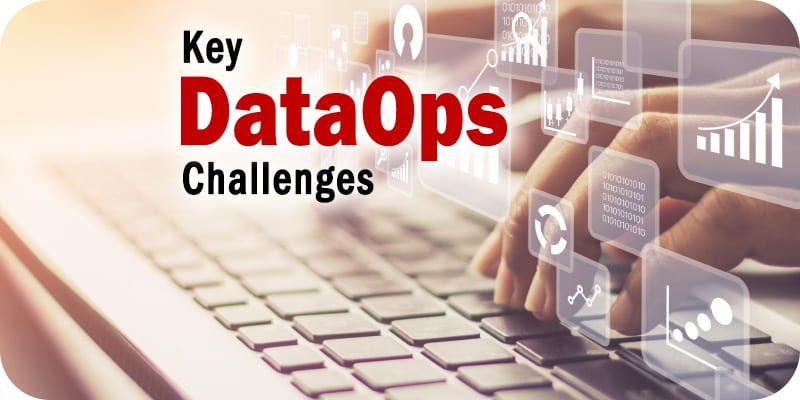
1. Complexity and Shifting Requirements – The data environment is the organization’s Wild West. Stakeholders struggling with shifting requirements and unforeseen changes to increasingly complex data pipelines. Complexity with limited visibility and poorly communicated changes to requirements give rise to data events, decreasing trust in data, and decreased performance and agility of data teams.”
2. Inconsistent Coordination – When working on multiple data projects involving different teams, it is essential to have strategic alignment. By streamlining procedures and setting up standards for communicating changes in advance, like agreeing upon approved and suitable data flows, teams can boost efficiency and avoid costly mistakes that slow everyone down.
3. Increasing Delays – Without observability across the pipeline at any given time, even minor unexpected blockage can hinder the efficiency of data operations. Speeding up and scaling processes without maintaining data quality isn’t workable either. Mapping their environment with data lineage permits teams to improvise pace and boost operational efficiency without compromising on data quality.
4. Lack of Automated Lineage Data – Every enterprise has ‘black boxes” hiding across countless databases, warehouses, processes, and BI tools involved in data operations. Using metadata to automate data pipelines, engineering teams can shift their aim away from manual, time-consuming tasks like adjusting models or transformations to make room for experimentation and innovation to happen.
5. Technical Data Lineage Tools – Today’s enterprise business users need lineage as much as their technical counterparts. Without business users’ ability to leverage self-servicing characteristics, the knowledge gap of data operations will continue to widen and hinder the company’s ability to compete on data-driven insights and business intelligence.
6. No Lineage – Without lineage to provide a verified source of truth for the end-user, the lack of trust in data keeps efficient data availability and data democratization out of reach for many.
Why is DataOps needed?
DataOps bridges the gap between those who collate the data, those who analyze the data, and those who will put the findings from that analysis to good use. The reason we need DataOps is a streamlined and effective process – is because time is of the essence in the world of business.
A few points to explain in brief the need for DataOps –
- Always more data… and more data sources
- Compliance is critical
- Business agility and responsiveness
- Too many data “tools”
- Data is precious
- The majority of processes are automated
- It extracts value from your data
- The process is adaptable and easy to maintain
- It encourages communication
What are the benefits of DataOps?
- Collaborative development – Collaborative development between business and data teams can enhance the agility of the business and avoid the business teams going and buying their own self-service tools, reinventing pipelines, and creating inconsistent processes that are tough to maintain.
- Increased efficiencies – Smaller teams with more powerful tools are faster and more productive. DataOps removes the need for bloated operational teams hand-cranking the management of development, test, and production infrastructure.
- Reduced implementation costs – By shortening the time to production and (where required) recovery, businesses can reduce costs by more than 70% – and that’s before we measure the added value from their data analytics.
- Maintainability and total cost of ownership – Don’t just think about the time and cost to implement a feature/requirement. Think, too, about the long-term support and maintainability of code and configuration. Think about the effort needed to perform routine tasks. DataOps streamlines all of these, so the Total Cost of Ownership (TCO) can be reduced by over 60%.
- Simplified orchestration and management – The DataOps philosophy transcends vendor-specific limitations. This allows your business to store all data together, creating more flexible, more useful use cases at a lower cost.
- Faster development – Increasing the agility of data processes will help gain access to valuable insights in hours or days rather than weeks and months
- Build once, reuse anywhere – Create logic as small, reusable components and then use/reuse many times. Avoid duplicated code that ends up inconsistent
- Data assurance – Improve the quality of the data you deliver to your business and provide assurances and guarantees your business stakeholders can rely on.
- Parallel development – Using technology to enhance collaboration allows data teams to do more – using DataOps we have seen a team of four people complete 200 cycles, 80 commits and 50 pushes to production in just one day.
- Improved supply chain – Establish a supply chain of data producers. Rather than treating a new data source as a one-time project, treat each department as a data user both producing and consuming data products for consumption across the data-driven enterprise.
Reference
- What is DevOps?
- DataOps Certifications
- DataOps Consultants
- DataOps Consulting Company
- Best DataOps Courses
- Best DataOps Tools
- Best DataOps Trainer
- Best DataOps Training
- Mastering Qualitative Research: The Role of Focus Groups in Data Collection - July 11, 2024
- What is robots ops? - July 10, 2024
- 5 Effective Online Learning Strategies for DevOps Professionals - July 4, 2024
Wow, thanks for the simple explanation and for making us understand the origin and development of DataOps
Nice explain of Evolution of DataOps, thanks for sharing
Thanks
thanks for explaining in such an easy way, one of the best explanation that I’ve found.
Thanks for sharing
Thank you for sharing this content. it is helpful
DataOps was first introduced by Lenny Liebmann, Contributing Editor, InformationWeek, in a blog post on the IBM Big Data & Analytics Hub titled “3 reasons why DataOps is essential for big data success” on June 19, 2014. The term DataOps was later popularized by Andy Palmer of Tamr and Steph Locke.